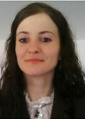
Laura Fanea
Cluj County Emergency Hospital, Romania
Title: Disease strategy management for future artificial intelligence-based ocular magnetic resonance imaging
Biography
Biography: Laura Fanea
Abstract
Statement of the Problem: Appropriate program implementation in ophthalmology is recommended to avoid, prevent or treat the socio-economic issues caused by blindness and visual impairment. Artificial Intelligence (AI) will help medical specialists evaluate at the highest possible standards any medical condition offering the most complex and accurate medical information in the most rapid way. The complex geometric-physicochemical quantitative information offered by Magnetic Resonance Imaging (MRI) on the qualitative anatomical biophysiology and/or pathophysiology of the eye covers the main eye structures: Cornea, aqueous humor, ciliary body, lens, vitreous humor, sclera, optic nerve and three retinal layers. Implementation of AI-based MRI techniques in ophthalmology could, therefore, play a crucial role in reducing the present socio-economic burden caused by eye disease.
Methodology & Theoretical Orientation: A Control/Normal (CN) database consisting of the already published qualitative and quantitative human ocular MRI information at 1, 1.5, and 3 T was used. Disease-Affected (DA) human eye regions were assessed by Relative-to-Normal (RN) values of each MRI parameter, calculated using the equation below: RN Parameter (%) = 100*(ParameterDA- ParameterCN)/(ParameterCN). Significance was considered for RN values >50%.
Findings: Detailed MRI physio-anatomical characteristics were defined based on the analyses of the MRI parameters evaluated. The complex disease staging scale was built using the relative-to-normal values calculated for the normal subjects and the MRI physio-anatomy defined. A scenario for AI-based ocular MRI is also presented.
Conclusion & Significance: The template for AI-based ocular MRI developed in this study can be immediately implemented in diagnostic ophthalmology and/or radiology. Future possible studies can extrapolate results in this study for AI-based medical diagnosis of any other organ in the human body, but also for AI-based medical prognosis, treatment and/or surgery.